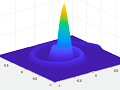
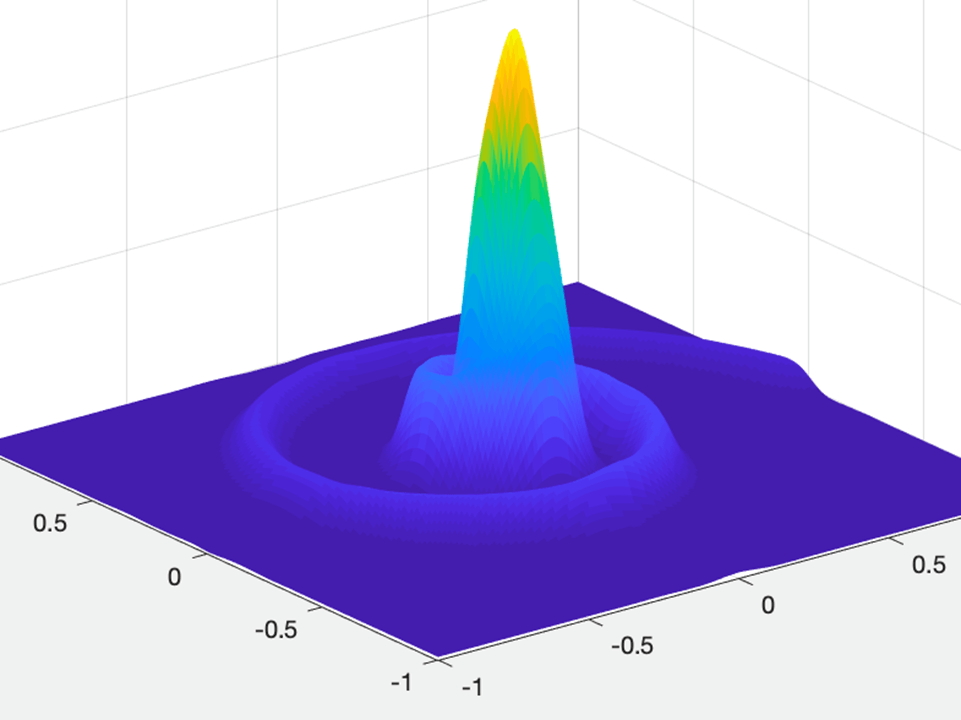
Occupation kernels and Liouville operators
Operator theoretic methods for data-driven identification, verification, and control synthesis.
Details and publicationsOperator theoretic methods for data-driven identification, verification, and control synthesis.
Details and publicationsCapitalizing on recent developments in reinforcement learning in continuous time and space, we develop novel model-based reinforcement learning methods that vastly improve data efficiency and usefulness for online optimal feedback control.
Details and publicationsMotivated by the need to adapt to changing models and reduce modeling errors in model-based control, we developed data-driven adaptive system identification methods to identify unknown parameters in the system model.
Details and publicationsBased on the premise that a cost (or reward) function fully characterizes the intent of the demonstrator, we developed methods to learn the cost function from observations for linear and nonlinear uncertain systems.
Details and publicationsExtensions of the LaSalle-Yoshizawa Theorem to nonsmooth nonautonomous systems and switched systems utilizing Filippov solutions of nonsmooth differential equations and nonstrict Lyapunov functions.
Details and publicationsPotential uses of neuromuscular electrical stimulation for gait modifications leading to management of Osteoarthritis related pain.
Details and publicationsData-driven model-based architectures to find feedback equilibrium solutions to differential games under relaxed excitation conditions.
Details and publicationsControl methods for systems with known and unknown state and input delays.
Details and publications